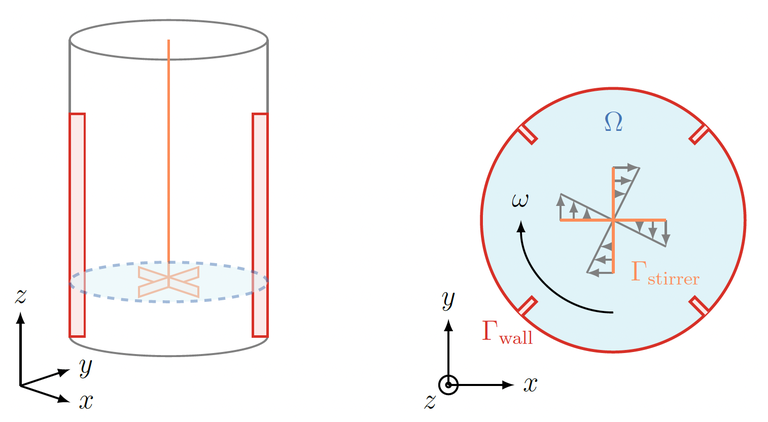
New publication uses PINNs to create a model hierarchy with differing fidelity
21 Mai 2024
We’re happy to announce that our new article entitled “A model hierarchy for predicting the flow in stirred tanks with physics-informed neural networks” has been published in the journal Advances in Computational Science and Engineering. In this collaborative work between the Institute for Mathematics and Computer-based Simulation of UniBw Munich, the Chair for Computational Analysis of Technical Systems of RWTH Aachen University, the Institute for Lightweight Design and Structural Biomechanics of TU Wien and the Institute of Bio- and Geosciences of the Research Center Jülich, the authors investigate the potential of creating a model hierarchy for predicting the flow field in stirred tank reactors with the help of physics-informed neural networks (PINNs).
Therefore, the authors explore different advanced techniques to enhance the accuracy of the fast-to-evaluate PINN models, including a formulation of the governing equations in polar coordinates, strong imposition of boundary conditions as well as a domain decomposition approach which allows to solve different equations in different parts of the computational domain. The best performing model is subsequently chosen for parameterization, yielding a surrogate model which is capable of predicting the flow features for a wide range of Reynolds numbers (up to 10,000).
Check out the early access to our publication below:
Veronika Trávníková, Daniel Wolff, Nico Dirkes, Stefanie Elgeti, Eric von Lieres, Marek Behr. A model hierarchy for predicting the flow in stirred tanks with physics-informed neural networks. Advances in Computational Science and Engineering. doi: 10.3934/acse.2024007