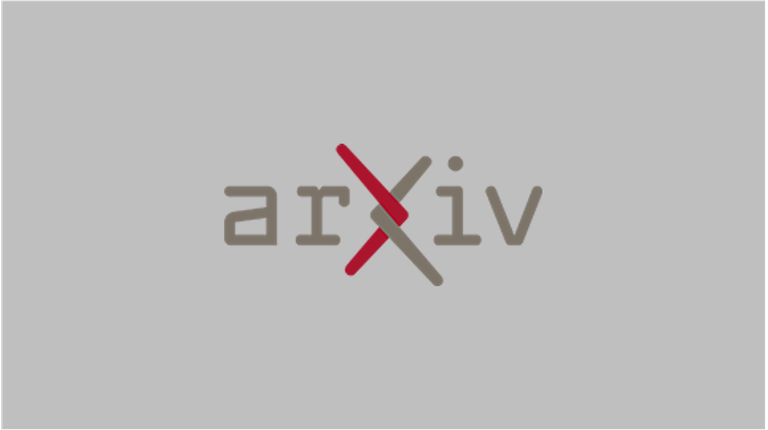
New preprint on physics-informed neural networks as surrogate model
10 Juni 2024
This study examines fast-to-evaluate surrogate modeling techniques for creating a hybrid digital twin of a steel-reinforced concrete beam. Two physics-informed neural network models are developed, integrating experimental data from a four-point bending test with physical laws.
The first model predicts time-dependent strains at fixed locations, inspired by a harmonic oscillator. It is compared to a purely data-driven method, highlighting the importance of physical laws for model extrapolation with limited data. This model also helps identify the system's natural frequency as an inverse solver.
The second model predicts strain distribution at a fixed time using sensor data and linear elasticity equations, emphasizing the importance of balancing loss components through appropriate loss weights.
Sahin, T., Wolff, D., von Danwitz, M., Popp, A. (2024). Towards a Hybrid Digital Twin: Physics-Informed Neural Networks as Surrogate Model of a Reinforced Concrete Beam. Preprint, submitted for publication arXiv